可扩展的神经学习排序 (LTR) 模型
import tensorflow as tf import tensorflow_datasets as tfds import tensorflow_ranking as tfr # Prep data ds = tfds.load("mslr_web/10k_fold1", split="train") ds = ds.map(lambda feature_map: { "_mask": tf.ones_like(feature_map["label"], dtype=tf.bool), **feature_map }) ds = ds.shuffle(buffer_size=1000).padded_batch(batch_size=32) ds = ds.map(lambda feature_map: ( feature_map, tf.where(feature_map["_mask"], feature_map.pop("label"), -1.))) # Create a model inputs = { "float_features": tf.keras.Input(shape=(None, 136), dtype=tf.float32) } norm_inputs = [tf.keras.layers.BatchNormalization()(x) for x in inputs.values()] x = tf.concat(norm_inputs, axis=-1) for layer_width in [128, 64, 32]: x = tf.keras.layers.Dense(units=layer_width)(x) x = tf.keras.layers.Activation(activation=tf.nn.relu)(x) scores = tf.squeeze(tf.keras.layers.Dense(units=1)(x), axis=-1) # Compile and train model = tf.keras.Model(inputs=inputs, outputs=scores) model.compile( optimizer=tf.keras.optimizers.Adam(learning_rate=0.01), loss=tfr.keras.losses.SoftmaxLoss(), metrics=tfr.keras.metrics.get("ndcg", topn=5, name="NDCG@5")) model.fit(ds, epochs=3)在 笔记本 中运行
TensorFlow 排序是一个开源库,用于开发可扩展的神经 学习排序 (LTR) 模型。排序模型通常用于搜索和推荐系统,但也已成功应用于各种领域,包括 机器翻译、对话系统 电子商务、SAT 求解器、智慧城市规划,甚至 计算生物学。
排序模型接受一个项目列表(网页、文档、产品、电影等),并生成一个优化排序的列表,例如最相关的项目位于顶部,最不相关的项目位于底部,通常是响应用户查询。
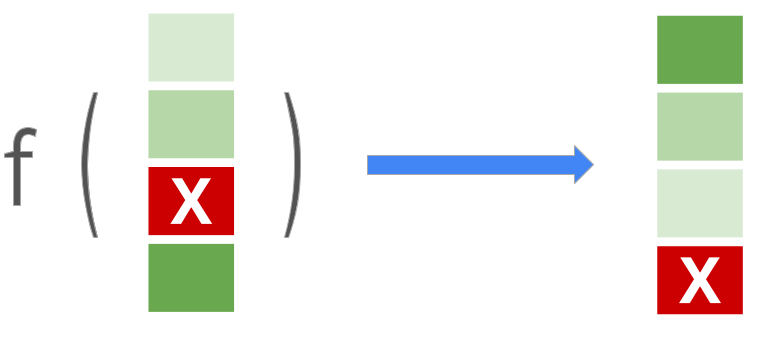
此库支持 LTR 模型的标准点式、成对式和列表式损失函数。它还支持各种排序指标,包括 平均倒数秩 (MRR) 和 归一化折损累计增益 (NDCG),因此您可以评估和比较这些方法以用于您的排序任务。排序库还提供用于增强排序方法的功能,这些方法由 Google 的机器学习工程师进行研究、测试和构建。
通过查看 教程 开始使用 TensorFlow 排序库。通过阅读 概述 了解有关库功能的更多信息。查看 TensorFlow 排序在 GitHub 上的源代码。